Predicting the efficacy of carbon reduction strategies By using deep learning methods, we have predicted carbon reduction and other climate-related outcomes with much faster turnaround times, and similar or better predictive accuracy, than using traditional physical simulation models. For example, with the University of Tokyo, we developed a neural network-based model that can simulate physical and chemical processes critical to carbon storage, such as the formation of hydrates that can fill cracks between sand and rocks under the ocean floor, thus playing a critical role in ensuring safer thousand-year storage of carbon. With this model, we were able to accurately predict hydrate formation 1,000 times faster than with numerical models. With Tsinghua University, we developed a neural network-based atmosphere simulator that estimates carbon and other pollutant emissions with better accuracy than widely used numerical atmosphere models, while using only 1 percent of the computational cost. For simulations of the behavior of fluids in the atmosphere to better understand the diffusion of pollutants and the global warming effect, we invented a neural network-based model to solve the necessary partial differential equations, delivering accuracy on par with traditional numerical solvers while delivering results 50 to 100 times faster. 8 Solving solar manufacturing problems before they start Satisfying the growing global demand for renewable energy requires reducing costs and better integrating renewables into electricity and transportation grids. In collaboration with one of India’s largest solar panel manufacturers, Microsoft researchers developed a machine learning-based computer vision system that can accurately detect and classify defects in solar panels as they are being manufactured. By fixing defects in real time, solar panel manufacturers can achieve higher yield and lower production costs, and solar energy operators can produce more energy per panel. Enabling systems change (continued) Supporting new carbon reduction roadmaps Carbon Insights is a visualization and analysis platform that provides carbon neutralization roadmaps for researchers, policy audiences, and other sustainability decision-makers. Dashboards and maps combine local detail with global consistency to show daily carbon emission sources, sinks, and flux with near-global coverage. The tool is based on data aggregated from Tsinghua University research and public datasets. It provides strategic insights for challenges such as prioritizing local emissions reductions or estimating impacts on economic growth. 1% We developed a neural network- based atmosphere simulator that estimates carbon and other pollutant emissions with better accuracy than widely used numerical atmosphere models, while using only 1 percent of the computational cost. Deep learning to predict carbon reduction and other climate-related outcomes. 35
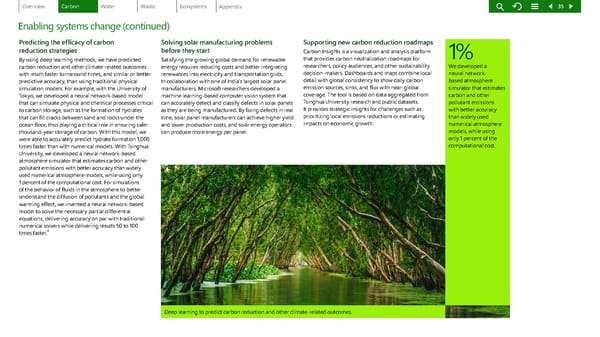