Data Labeling and Augmentation Job Attributes We annotated jobs in two major ways: ● Company attributes:In this analysis, we segmented companies based on various attributes. These were based on our own internal research and classification of companies and did not correspond to government agency mappings because this was a global dataset. These are further described in the glossary. ● Job attributes: We defined attributes of jobs using our proprietary job taxonomy. Our taxonomy does not correspond to government agency mappings (e.g., the Bureau of Labor Statistics Standard Occupational Classification) or other industry mappings. An example of an attribute we defined is whether a job is a remote position or not. We used a variety of contextual information including job titles, locations, and job post content in addition to linguistic attributes to determine whether a role was truly remote. This allowed us to annotate remote jobs with a high fidelity and exclude jobs such as those that require experience with “remote sensing” or “remote services” or that are temporarily remote. Applicant Attributes We annotated our job outcomes data in three additional ways: 1. Application sources: a. ATSs define application sources in a variety of different ways. Some enable user-inputted data, which is where we get ‘LinkedIn,’ ‘Linked-in,’ ‘linkedin,’ and other ways to denote LinkedIn. Meanwhile, others define whether a source like LinkedIn is being used as a sourcing/prospecting tool or as a job board. b. Our platform organizes over 300,000 application sources into core categories. In this report, we present a top-level grouping focused on whether applicants applied through inbound or outbound sources. These categories are further specified in the glossary. 2. Applicant gender representation: a. We analyze applicant pool attributes in the context of candidate gender inferences. These were based on our proprietary inference engine. b. Comparisons against Equal Employment Opportunity (EEO) self-reports suggest this is 94% accurate and does not suffer from non-response issues associated with EEO data. 3. Data hygiene: a. Because we analyzed applicant pool sizes, we excluded any recruiting data hygiene issues that would skew our ability to define the average applicant pool size/makeup © Tapwage Inc 2022 30
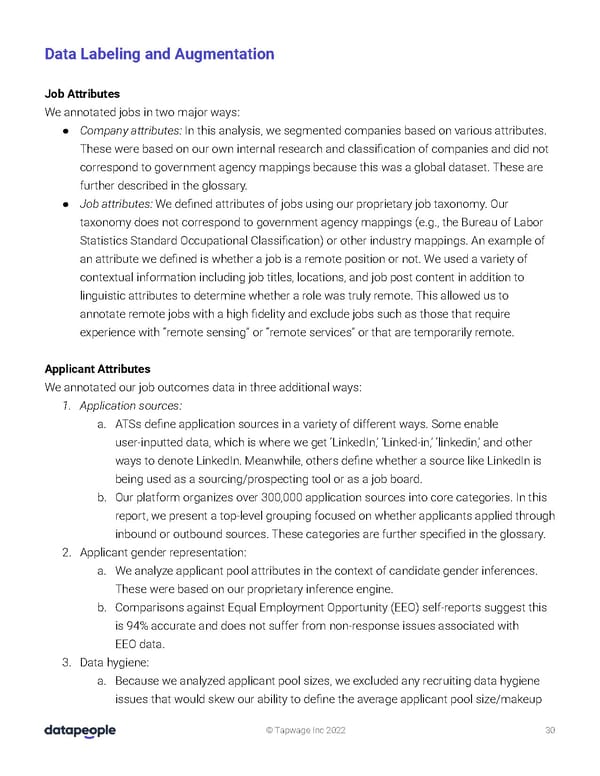