DATA SCIENCE INTELLIGENT CAPTURE USINGCONFIDENCE SCORES Once we understand field-level confidence scores, we can measure and tune field-level accuracyfor higher quality data results. To be effective and reliable, this confidence score analysis should be based on several hundred to several thousand samples, ensuring the analysis includes the broadest array of variances in document quality and layout. In the above example, the information we are concerned with are the last three columns: the first two are the transcription of the field (date of birth) from the OCR engine and the confidence score for that field (also generated by the OCR engine). The final columnshowsthe result from analysis astowhethertheOCRanswermatchesexactlywhatisonthedocument image.Oncethisisdone,answerscanbe ordered by confidence score from high to low in order to identify the optimal threshold. To find the optimal threshold, you must calculate accuracy provided at a specified threshold. We measure actual accuracyof a specified thresholdby dividing the numberof OCR answers abovethe thresholdthat are accurate by all answers provided above the threshold. In this scenario, all but one answer with a score equal to or above 74 are correct. There is also an answer below the threshold of 74 that is correct. Therefore, the majority of data can be segmentedinto two groups: one with a field-level confidence score of 74 or above and one group with scores of less than 74. Separation of data into these two groups is the goal of using confidence scores.
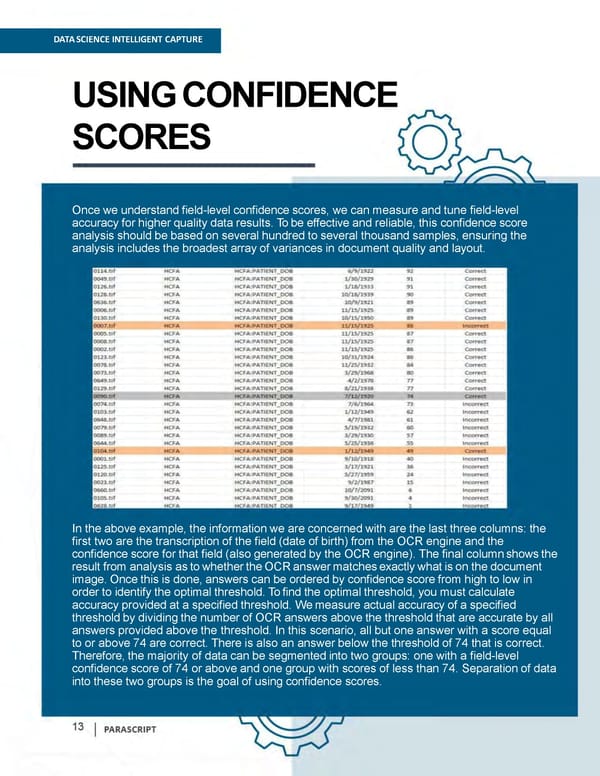