Preqin ESG Solutions 33 b. Utilized this data to create a single score for each SASB factor, encapsulating risk exposure across all the countries and dependencies. To assure that our geographic model is conformable, we used the same factors as in the industry risk model. Since no third party provides SASB risk factors at country level, these scores were calculated internally by the ESG team. 2. Then, for each country or dependency (in ISO3 taxonomy), we: a. Converted these scores into numeric values between 0.0 and 10.0. b. Conducted a sequence of aggregations to generate 35 Geography Risk Scores across SASB factor, SASB pillar, E/S/G and overall ESG levels. The highest tier estimate gave us the 昀椀nal risk score at the country level. 3. To make use of these results within our system, we mapped ISO3 codes to Preqin country classi昀椀cations. 4. Every portfolio company on Preqin Pro is classi昀椀ed as having its headquarters in a single country, so we were able to assign a Geography Risk Score to each company with identi昀椀ed headquarters. The composition of our geographic dataset mirrors, to a considerable extent, the inputs used by other public ratings providers that consider ESG risk rigorously. For instance, while there is no codi昀椀ed metric measuring “Human Rights & Community Relations” risk exposure at the country level, academics have amassed an array of data points relevant to its evaluation, including air and water quality, access to nutrition, labor rights and healthcare access, modern slavery, and poverty ratios. Other seemingly viable variables were disregarded due to narrow country coverage, quality issues and cross-correlation with other data. ↗ Back to Contents
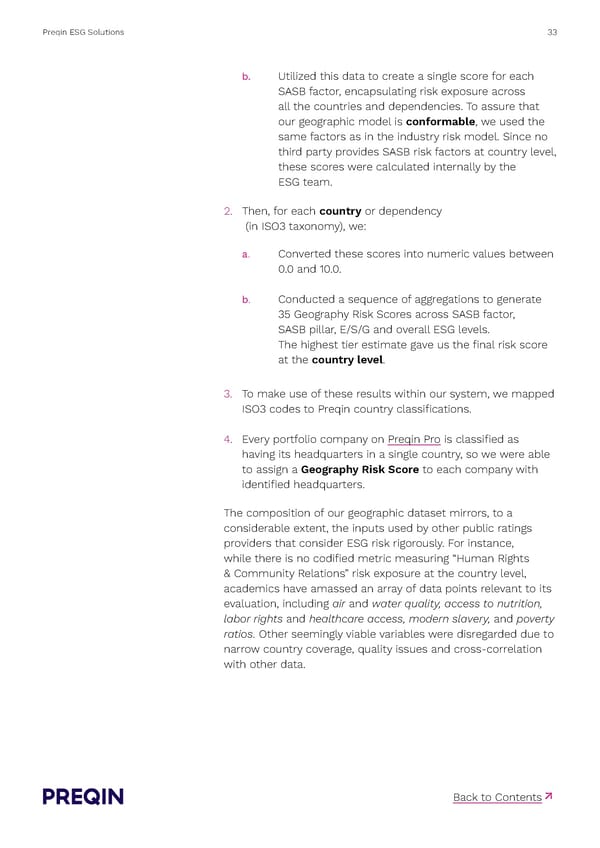