Preqin ESG Solutions 58 iii. The sum in (dii) is our similarity score for a given private company. A company with no matching stemmed keywords in its description receives no score. e. We then sort all the private companies (in descending order, including the ones already tagged as a “Very Likely” source of impact) according to their similarity with SDG objectives. f. For each private company on our platform without a likelihood level rating: i. If it lies in a top quartile of similarity scores with SDG corpus, it is assessed as a “Likely” source of impact potential ii. If it lies in the second quartile its impact potential is assessed as “Possible” iii. Otherwise, it is tagged as “Unlikely/Unknown”. g. We then repeat points a to c with EU Taxonomy as a corpus, using the outputs to repeat points (d-f) for each infrastructure asset. Note that beyond Step 2 no asset is ever tagged as a “Very Likely” source of impact, no matter how corpus-aligned its description is. This is an intentionally conservative choice, to mitigate potential greenwashing in the asset’s description. It also enables our clients to distinguish our estimates from the data. We will illustrate this approach using a description of a company that is neither held by an impact fund, nor it is operating in an impact-related industry. While its impact potential cannot be assessed in the 昀椀rst two steps of our procedure, the company’s description contains multiple SDG keywords. ↗ Back to Contents
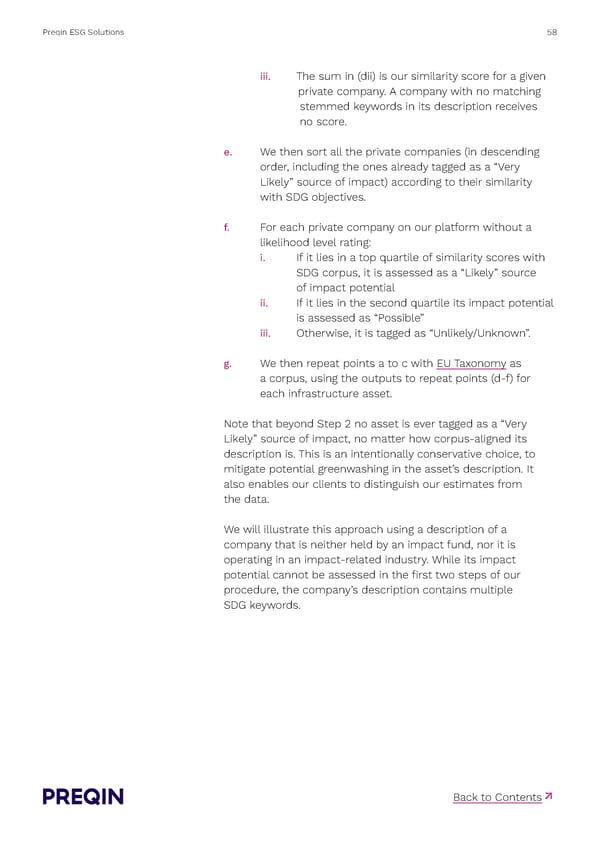