DATA SCIENCE INTELLIGENT CAPTURE IDENTIFYINGGOODDATA FROM BAD DATA Once we have gathered a good sample set and configured the software, it is time to evaluate the results in order to optimize the system. Our sample data along with the “answer key” allow us to compare the results of the system to the correct answer. This allows us to calculate the read rate for each field. We also spend a lot of time analyzing another number called the confidencescore. If you are a technical person who has worked with OCR software, then you probably have heard and even made use of a confidence score. All OCR software provides character-levelandword-levelconfidencescores.These scoresprovidethedeveloper an indication of whether the OCR software finds the answer to be correct. The scores are not representative of probabilities so a score of 80 does not mean an 80% probability of being correct. Thesecharacter/word scorescanbeuseful. However, whenitcomestoactual dataextraction—notsimply converting an image to text—another confidence score comes into play, the data field confidence score. Just like page-level OCR, software focused on data extraction produces the field confidencescore. FormXtra.AIisfocusedonfield-leveldatalocationandextractionwhichdiffers frommore genericfull-pageOCRsoftwaresuchasABBYYFinereader,Nuance OmniPageSDKor OCR available through Google, Amazon and Microsoft. The field-level confidence score uses the raw OCR characterand word-level scores andsynthesizesthemwith other available information to arrive at a final score produced by the software. This other information can be a data type (e.g., numeric, letters), format (e.g., phonenumbervs.creditcardnumber),etc.Whenitcomestoachievingtrue automation,theseconfidencescoresarecritical.Unfortunately,mostsolutions cannot supporttrue automation. To understand why and the potential significant negative impact on your project, read on.
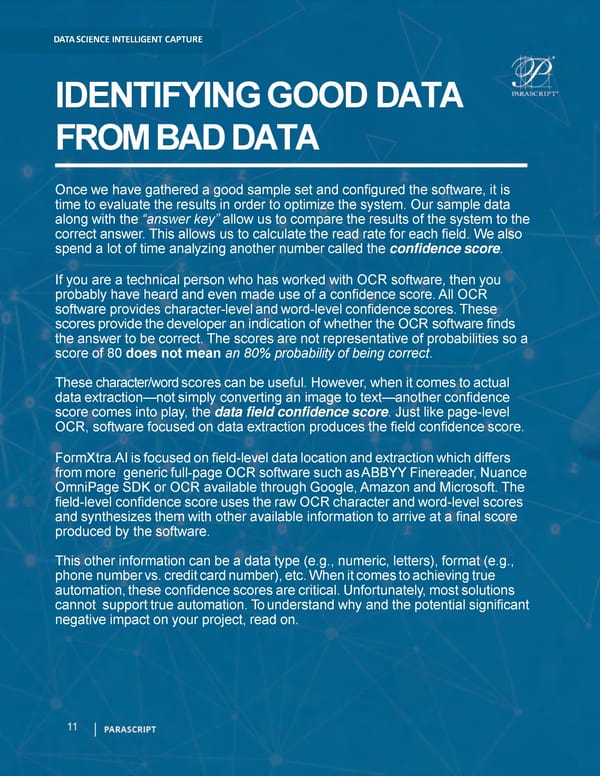