34 Enabling systems change We know that our action alone will not address the climate crisis. We are focused on new ways to harness the power of technology, partnerships, investments, and policy to drive impact at scale and pace to help the world get to carbon zero. At Microsoft we know that our action alone will not address the climate crisis, so we must help develop the markets for carbon abatement approaches. This is a partnership between our operational sustainability program, Climate Innovation Fund, and Microsoft Research to ensure that we are not only procuring and funding the technologies we need to meet our commitments, but also investing in the companies that provide them—and supporting research and development for pre-commercial solutions. Research and development Researchers at Microsoft tackle critical challenges that will shape the future of the world and the company. Given the significa nce of sustainability challenges for our global future, r esearch teams around the world are developing new technologies and approaches to reduce carbon, use comput e resources more efficiently, and bring AI to bear on pro blems from carbon emissions modeling to improvements in renewable energy. Improving reliability of carbon removal projects Afforestation (growth of net new trees) pulls carbon from the atmosphere. Trees on farms can also improve agricultural productivity and provide additional income to farmers through the sale of carbon credits. Buyers of such credits, however, want to ensure that the farmer is properly incentivized to safeguard tree growth over time. Using the lens of contract theory, Microsoft research shows how to calculate the optimal stream of payments over time to ensure incentive alignment. This research can be applied to enlarge the supply of carbon reduction credits, especially outside the developed world. Trees on farms can provide additional income to farmers through the sale of carbon credits. Using contract theory, Microsoft research shows how to calculate the optimal stream of payments to ensure farmers are properly incentivized to safeguard tree growth over time. Reducing the energy impact of AI Many AI tasks, like predicting the next word a person will type, rely on machine learning with neural networks, also known as deep learning. These neural networks can unlock new sustainability solutions, but also can consume a lot of energy. In a new effort, we are building tools for AI developers to predict the energy consumption of a neural network before deployment, so that more compute - and energy-efficient networks can be designed upf ront. We have progressed on other techniques enab ling more efficient networks, including factorizable neu ral operators (FNO), a compression technique that affords reductions in compute and memory requirements. Applied to large-scale language models such as BERT, FNO reduced memory usage by 80 percent and prediction time by 50 percent, with less than a 5 percent reduction in accuracy. Further improvements may be achieved by combining FNO with other model compression methods, such as distillation and quantization. Collaborating with researchers at NVIDIA, we’ve developed a new method to find neural netwo rks that satisfy hardware constraints, such as low power consumption for mobile devices. This highly efficie nt method can find high-performing architectures i n minutes, enabling carbon savings compared to previous methods. We also advanced AI systems research to explicitly optimize for server power reduction, in add ition to capacity efficiency; this work has resulted in a mult i-GWh/year reduction of power consumption for Azure and Microsoft 365.
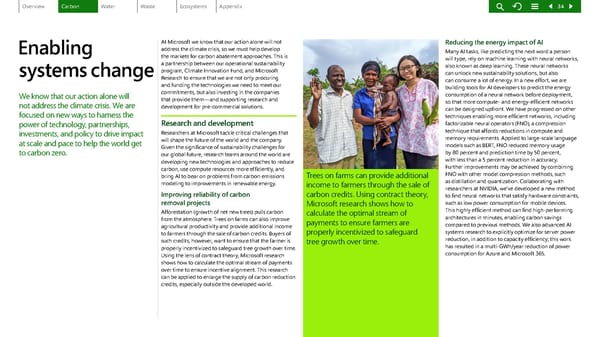